Virtual Registration Package
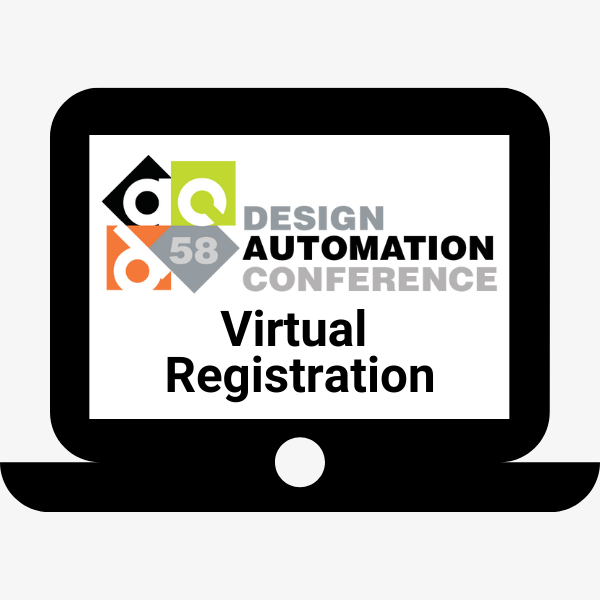
DAC 58 will bring together the most forward-thinking leaders in design automation, representing both industry and academia, to drive innovation and research in the field of design and automation of electronic systems. We understand there are barriers to travel outside of your control, so if you are unable to join your peers and colleagues for the premier conference in this industry, we want to ensure you are still able to access the cutting-edge research you need to stay ahead of the competition and on the forefront of electronic design. Take advantage of the exclusive DAC Virtual Registration Package which will grant you access to a select number of sessions along with limited networking capabilities on our virtual platform from the comfort of your own home or office.
Register Now
View the Registration Package Options
*If you've previously registered for 58th DAC, please use the guide below for information of what's included in your registration.
.png?ver=Epx1mVy_emQz2bFl4yQ7mg%3d%3d)
58th DAC Keynote Speakers
The Potential of Machine Learning for Hardware Design
In this talk I'll describe the tremendous progress in machine learning over the last decade, how this has changed the hardware we want to build for performing such computations, and describe some of the areas of potential for using machine learning to help with some difficult problems in computer hardware design. I'll also briefly touch on some future directions for machine learning and how this might affect things in the future.
GPUs, Machine Learning, and EDA
GPU-accelerated computing and machine learning (ML) have revolutionized computer graphics, computer vision, speech recognition, and natural language processing. We expect ML and GPU-accelerated computing will also transform EDA software and as a result, chip design workflows. Recent research shows that orders of magnitudes of speedups are possible with accelerated computing platforms and that the combination of GPUs and ML can enable automation on tasks previously seen as intractable or too difficult to automate. This talk will cover near-term applications of GPUs and ML to EDA tools and chip design as well as a long term vision of what is possible. The talk will also cover advances in GPUs and ML-hardware that are enabling this revolution.
When the Winds of Change Blow, Some People Build Walls and Others Build Windmills
Mr. Costello is considered to have founded the EDA industry when in the late 1980s he became President of Cadence Design Systems and drove annual revenues to over $1B—the first EDA company to achieve that milestone. In 2004, he was awarded the Phil Kaufman Award by the Electronic System Design Alliance in recognition of his business contributions that helped grow the EDA industry. After leaving Cadence, Joe has led numerous startups to successful exits such as Enlighted, Orb Networks, think3, and Altius. He received his BS in Physics from the Harvey Mudd College and also has a master's degree in Physics from both Yale University and UC Berkeley.
AI, Machine Learning, Deep Learning: Where are the Real Opportunities for the EDA Industry?
Learn More
58th DAC SkyTalk Speakers
Cloud & AI Technologies for Faster, Secure Semiconductor Supply Chains
Semiconductors are deeply embedded in every aspect of our lives, and recent security threats and global supply chain challenges have put a spotlight on the industry. Significant investments are being made both by nation states and commercial industry, to manage supply chain dependencies, ensure integrity and build secure, collaborative environments to foster growth. These shifts provide unique opportunities for our industry. This talk blends insights and experiences from government initiatives and Azure's Special Capabilities & Infrastructure programs, to outline how Cloud + AI technologies, along with tool vendors, fabless semiconductor companies, IP providers, foundries, equipment manufacturers and other ecosystem stakeholders can contribute to building a robust, end-to-end, secure silicon supply chain for both commercial and government applications, while generating value for their businesses.
The precision scaling powered performance roadmap for AI Inference and Training systems
Over the past decade, Deep Neural Network (DNN) workloads have dramatically increased the computational requirements of AI Training and Inference systems - significantly outpacing the performance gains obtained traditionally using Moore's law of silicon scaling. New computer architectures, powered by low precision arithmetic engines (FP16 for training and INT8 for Inference), have laid the foundation for high performance AI systems - however, there remains an insatiable desire for AI compute with much higher power-efficiency and performance. In this talk, I'll outline some of the exciting innovations as well as key technical challenges - that can enable systems with aggressively scaled precision for inference and training, while fully preserving model fidelity. I'll also highlight some key complementary trends, including 3D stacking, sparsity and analog computing, that can enable dramatic growth in the AI system capabilities over the next decade.
Cross-Disciplinary Innovations Required for the Future of Computing
With traditional drivers of compute performance a thing of the past, innovative engineers are tapping into new vectors of improvement to meet the world's demand for computation. Like never before, the future of computing will be owned by those who can optimize across the previously siloed domains of silicon design, processor architecture, package technology and software algorithms to deliver performance gains with new capabilities. These approaches will derive performance and power efficiency through tailoring of the architecture to particular workloads and market segments, leveraging the much greater performance/Watt and performance/area of accelerated solutions. Designing and verifying multiple tailored solutions for markets where a less efficient general purpose design formerly sufficed can be accomplished through modular architectures using 2.5D and 3D packaging approaches. Delivering on modular solutions for high volume markets requires simultaneously optimizing across packaging, silicon, interconnect technologies where in the past, silicon design was sufficient. This talk will cover these trends with the vectors of innovation required to deliver these next generation compute platforms.
Learn More
58th DAC TechTalk Speakers
Reimagining Digital Simulation
In the last few decades, digital event-driven simulation has largely relied on underlying hardware for performance gains; core algorithms have not undergone truly transformative changes. Past efforts to accelerate simulation with special purpose hardware has repeatedly fallen behind the ever-improving performance of general-purpose computers, enabled by Moore's Law. Emulation-based strategies have also reached a performance ceiling. We are now at the end of the road with Moore's Law, and the time is right to fundamentally rethink simulation algorithms, methodologies, and computational strategies: considering hyperscaling, facilitated by the cloud, and advances in domain specific computing. This talk will examine the past and a possible future of simulation, a key technology enabler for advanced chip designs.
Delivering Systemic Innovation to Power in the Era of SysMoore
The SysMoore era is characterized by the widening gap between what is realized through classic Moore's Law scaling and massively increasing system complexity. The days of traditional System-on-a-chip complexity are giving way to systems-of-chips complexity, with the continued need for smaller, faster, and lower-power process nodes coupled with large-scale multi-die integration methodologies to coalesce new breeds of intelligence and compute, at scale. To enable such systems, we need to look beyond targeted but piece-meal innovation to something much broader and more able to deliver holistically and on a grander scale.
Systemic thinking coupled with systemic innovation is key to addressing both prevailing and future industry challenges and approaching them comprehensively is necessary to deliver the technological and productivity gains demanded to drive the next wave of transformative products.
This presentation will outline some of the myriad prevailing challenges facing designers in this era of SysMoore and the systemic innovations across the broad, silicon-to-software spectrum to address them. Join us to learn, how a combination of intelligent, autonomous, and analytics-driven design, is paving the way to reliable, autonomous, always-connected vehicles and how this hyper-integrated approach to innovation is being deployed to deliver the secure, AI-enabled, multi-die HPC compute systems of tomorrow. And much more!
More than Moore and Charting the Path Beyond 3nm
For more than fifty years, the trend known as Moore’s Law has astutely predicted a doubling of transistor count every twenty-four months. As 3nm technology moves into production, process engineers are feverishly working to uphold Moore’s Law by further miniaturizing the next generation of semiconductor technology. Meanwhile, a second trend referred to as “More than Moore” was coined in 2010 to reflect the integration of diverse functions and subsystems in 2D SoCs and 2.5D and 3D packages. Today, the trends of Moore’s Law and “More than Moore” synergize to produce ever higher value systems.
Working together, advances in both process technology and electronic design automation (EDA) have driven fundamental evolutions behind these two important semiconductor trends. This talk will examine the amazing and innovative developments in EDA over the years, culminating in the era of 3DIC and Machine Learning-based EDA to chart the path to 3nm and More than Moore.
The AI Hype Cycle is Over. Now What?
The expectations around AI and ML have been enormous, which fueled investment and innovation as companies scrambled for scalable approaches to building and deploying AI and ML solutions. Experimentation, in both hardware and software, has been the order of the day:
- Ramping up the core technology to improve accuracy and take on more use cases.
- Experimenting with the technology (models and processors) to understand what was possible, what worked, what didn't and why.
The exuberance of the moment, however, created some unintended consequences. Take, for example, a fully parameterized, complex Transformer network. In an analysis by Northeastern University, the 300 million parameter model took 300 tons of carbon to train. Since then, accuracy and efficiency have improved gradually.
Today, as the shouting dies down, the biggest trend – one that is having profound effects in helping teams innovate – is around hardware. The days of general-purpose hardware anchoring AI and ML are quickly giving way to specialized compute that allows engineers to not only tune their solutions for accuracy and efficiency but deploy their solutions more effectively across the compute spectrum. Industry veteran Steve Roddy, head of AI and ML product for Arm, will describe how a new era of democratized design is accelerating innovation in AI and design teams who embrace are speeding ahead of the pack.
Learn More
Virtual Sessions
Architecture-Aware Precision Tuning with Multiple Number Representation Systems*
Authors:
- Daniele Cattaneo, Politecnico di Milano, Milan, Italy
- Michele Chiari, Politecnico di Milano, Milan, Italy
- Nicola Fossati, Politecnico di Milano, Milan, Italy
- Giovanni Agosta, Politecnico di Milano, Milan, Italy
- Stefano Cherubin, Codeplay Software Ltd., Edinburgh, United Kingdom
Description: Precision tuning trades accuracy for speed and energy savings, usually by reducing the data width, or by switching from floating point to fixed point representations. However, comparing the precision across different representations is a difficult task. We present a metric that enables this comparison, and employ it to build a methodology based on Integer Linear Programming for tuning the data type selection. We apply the proposed metric and methodology to a range of processors, demonstrating an improvement in performance (up to 9x) with a very limited precision loss (<2.8% for 90% of the benchmarks) on the PolyBench benchmark suite.
Distilling Arbitration Logic from Traces using Machine Learning: A Case Study on NoC*
Authors:
- Yuan Zhou, Zhiru Zhang, Cornell University, Ithaca, NY
- Hanyu Wang, Shanghai Jiao Tong University, Shanghai, China
- Jieming Yin, Lehigh University, Bethlehem, PA
Description:
Deep learning techniques have been shown to achieve superior performance on several arbitration tasks in computer hardware. However, these techniques cannot be directly implemented in hardware because of the prohibitive area and latency overhead. In this work, we propose a novel methodology to automatically "distill" the arbitration logic from simulation traces. We leverage tree-based models as a bridge to convert deep learning models to logic, and present a case study on a network-on-chip port arbitration task. The generated arbitration logic achieves significant reduction in average packet latency compared with the baselines.
DNN-Opt: An RL Inspired Optimization for Analog Circuit Sizing Using Deep Neural Networks
Authors:
- Ahmet F. Budak, The University of Texas at Austin, Austin, TX
- David Pan, The University of Texas at Austin, Austin, TX
- Nan Sun, The University of Texas at Austin, Austin, TX
- Prateek Bhansali, Intel Corporation, Hillsboro, OR
- Chandramouli V. Kashyap, Intel Corporation, Hillsboro, OR
- Bo Liu, University of Glasgow, Glasgow, United Kingdom
Description:
In this paper, we present DNN-Opt, a novel Deep Neural Network (DNN) based black-box optimization framework for analog sizing. Our method outperforms other black-box optimization methods on small building blocks and large industrial circuits with significantly fewer simulations and better performance. This paper's key contributions are a novel sample-efficient two-stage deep learning optimization framework inspired by the actor-critic algorithms developed in the Reinforcement Learning (RL) community and its extension for industrial-scale circuits. This is the first application of DNN based circuit sizing on industrial scale circuits to the best of our knowledge.
Gemmini: Enabling Systematic Deep-Learning Architecture Evaluatioin via Full-Stack Integration
Authors:
- Hasan N. Genc, University of California, Berkeley, Berkeley, CA
- Seah Kim, University of California, Berkeley, Berkeley, CA
- Alon Amid, University of California, Berkeley, Berkeley, CA
- Ameer Haj-Ali, University of California, Berkeley, Berkeley, CA
- Vighnesh Iyer, University of California, Berkeley, Berkeley, CA
- Pranav Prakash, University of California, Berkeley, Berkeley, CA
- Jerry Zhao, University of California, Berkeley, Berkeley, CA
- Daniel Grubb, University of California, Berkeley, Berkeley, CA
- Harrison Liew, University of California, Berkeley, Berkeley, CA
- Howard Mao, University of California, Berkeley, Berkeley, CA
- Albert Ou, University of California, Berkeley, Berkeley, CA
- Colin Schmidt, University of California, Berkeley, Berkeley, CA
- Samuel Steffl, University of California, Berkeley, Berkeley, CA
- John Wright, University of California, Berkeley, Berkeley, CA
- Ion Stoica, University of California, Berkeley, Berkeley, CA
- Krste Asanovic, University of California, Berkeley, Berkeley, CA
- Borivoje Nikolic, University of California, Berkeley, Berkeley, CA
- Yakun Sophia Shao, University of California, Berkeley, Berkeley, CA
- Jonathan Ragan-Kelley, Massachusetts Institute of Technology, Cambridge, MA
Description:
DNN accelerators are often developed and evaluated in isolation without considering the cross-stack, system-level effects in real-world environments. This makes it difficult to appreciate the impact of System-on-Chip (SoC) resource contention, OS overheads, and programming-stack inefficiencies on overall performance/energy-efficiency. To address this challenge, we present Gemmini, an open-source, full-stack DNN accelerator generator. Gemmini generates a wide design-space of efficient ASIC accelerators from a flexible architectural template, together with flexible programming stacks and full SoCs with shared resources that capture system-level effects. Gemmini-generated accelerators have also been fabricated, delivering up to three orders-of-magnitude speedups over high-performance CPUs on various DNN benchmarks.
A Resource Binding Approach to Logic Obfuscation
Authors:
- Michael Zuzak, University of Maryland, College Park, College Park, MD
- Yuntao Liu, University of Maryland, College Park, College Park, MD
- Ankur Srivastava, University of Maryland, College Park, College Park, MD
Description:
Logic locking counters security threats during IC fabrication. Research has identified a trade-off between 2 goals of locking, error injection and SAT attack resilience. As a result, locking often cannot inject sufficient error to impact an IC while maintaining SAT resilience. We propose using architectural context available during resource binding to co-design architectures/locking configurations with high corruption and SAT resilience. We propose 2 security-focused binding/locking algorithms and apply them to bind/lock 11 MediaBench benchmarks. These circuits showed a 26x and 99x increase in the application errors of a fixed locking configuration while maintaining SAT resilience and incurring minimal design overhead.
;
Accelerating EDA Algorithms with GPUs and Machine Learning
Topic Area(s): EDA, Machine Learning/AI
Session Organizers: Brucek Khailany, NVIDIA, Austin, TX; David Pan, The University of Texas at Austin, Austin, TX
Recent advancements in GPU accelerated computing platforms and machine learning (ML) based optimization techniques have led to exciting recent research progress with large speedups on many EDA algorithms fundamental to semiconductor design flows. In this session, we highlight ongoing research deploying GPUs and ML to mask synthesis, IC design automation, and PCB design at commercial EDA vendors and semiconductor design and manufacturing companies. Research into mask synthesis techniques shows the potential for GPUs to accelerate inverse lithography and for running training and inference of ML models for process modeling. In PCB layout editing, GPU-accelerated path rendering techniques can scale to millions of rendered objects with interactive responsiveness. In IC physical design, GPU-accelerated reinforcement learning for DRC fixing combined with traditional EDA optimization techniques can automate standard cell layout generation. The combination of GPUs and ML can enable large speedups and automate key EDA tasks previously seen as intractable.
Presentations include:
Democratizing Design Automation: Next Generation Opensource Tools for Hardware Specialization
Topic Area(s): EDA, Machine Learning/AI
Session Organizer: Antonino Tumeo, Pacific Northwest National Laboratory, Richland, WA
The growth of autonomous systems, coupled with design efforts and cost challenges brought by new technology nodes, is driving the need for generators that could quickly transition high-level algorithmic specifications to specialized hardware implementations. The necessity to explore additional dimensions of the design space (e.g., accuracy, security, system size and cooling) is further emphasizing the need for interoperable tools. This special session focuses on efforts for interoperable, modularized, and opensource tools to provide a no-human-in-the-loop design cycle from high-level specifications to ASICs and further promote novel research. The first talk introduces the status quo and CIRCT, an initiative aiming at applying MLIR and the LLVM development methodology to design automation. The second and third talks describe state-of-the-art tools, for high-level synthesis, and for logic synthesis, respectively, and discuss explorations to bridge the two. The session overviews how interoperability is achieved today, opportunities, challenges, and new perspectives enabled by community efforts.
Presentations include:
Design Automation of Autonomous Systems: State-of-the-Art and Future Directions
Topic Area(s): Autonomous Systems, Design
Session Organizer: Qi Zhu, Northwestern University, Evanston, IL
Shaoshan Liu, PerceptIn, Mountain View, CA
Design processes leverage various automated tools to support requirement engineering, design, implementation, verification, validation, testing and evaluation. In the domains of automotive and aerospace, design automation processes and tools have been architected and developed over the years and used to design products with established level of confidence. The recent success of Artificial Intelligence (AI) has shown great promises in improving system intelligence and autonomy for these applications. However, the adoption of those techniques also presents significant challenges for the design processes to ensure system safety, performance, reliability, security, etc. This special session will discuss essential design automation processes/tools and industrial efforts to support the development and deployment of future autonomous systems, particularly in the domains of automotive and aerospace.
Presentations include:
Hardware Aware Learning for Medical Image Computing and Computer Assisted Intervention
Topic Area(s): Design, Machine Learning/AI
Session Organizer: Lei Yang, University of New Mexico, Albuquerque, NM
Deep learning has recently demonstrated performance comparable with, and in some cases superior to, that of human experts in medical image computing. However, deep neural networks are typically very large, which combined with large medical image sizes create various hurdles towards their clinical applications. In medical image computing, not only accuracy but also latency and security are of primary concern, and the hardware platforms are sometimes resource-constrained. The first two talks in this session propose novel solutions for the data acquisition and data processing stages in medical image computing respectively, using hardware-oriented schemes for lower latency, memory footprint and higher performance in embedded platforms. Considering the privacy requirement, the third talk further demonstrates a software/hardware co-exploration framework for hybrid trusted execution environment in medical image computing, preserving privacy while achieving higher efficiency than human experts.
Presentations include:
Machine Learning Meets Computing Systems Design: The Bidirectional Highway
Topic Area(s): Design, Machine Learning/AI
Session Organizer: Partha P. Pande, Washington State University, Pullman, WA
With the rising needs of advanced algorithms for large-scale data analysis and data-driven discovery, and significant growth in emerging applications from the edge to the cloud, we need low-cost, high-performance, energy-efficient, and reliable computing systems targeted for these applications. Developing these application-specific hardware elements must become easy, inexpensive, and seamless to keep up with extremely rapid evolution of AI/ML algorithms and applications. Therefore, it is of high priority to create innovative design frameworks enabled by data analytics and machine learning that reduces the engineering cost and design time of application-specific hardware. There is also a need to continually advance software algorithms and frameworks to better cope with data available to platforms at multiple scales of complexity. To the best of our knowledge, this is the first special session at any EDA conference that explores both directions of cross-fertilization between computing system design and ML.
Presentations include:
A Quantum Leap in Machine Learning: From Applications to Implementations
Topic Area(s): Design
Session Organizer: Robert Wille, Johannes Kepler University, Linz, Austria
Classical machine learning techniques that have been extensively studied for discriminative and generative tasks are cumbersome and, in many applications, inefficient. They require millions of parameters and remain inadequate in modeling a target probability distribution. For example, computational approaches to accelerate drug discovery using machine learning face curse-of-dimensionality due to exploding number of constraints that need to be imposed using reinforcement learning. Quantum machine learning (QML) techniques, with strong expressive power, can learn richer representation of data with less number of parameters, training data and training time. However, the methodologies to design these QML workloads and their training is still emerging. Furthermore, usage model of the small and noisy quantum hardware in QML tasks to solve practically relevant problems is an active area of research. This special session will provide insights on building, training and exploiting scalable QML circuits to solve socially relevant combinatorial optimization applications including drug discovery.
Presentations include:
Smart Robots with Sensing, Understanding, and Acting
Topic Area(s): Autonomous Systems, Machine Learning/AI
Session Organizer: Janardhan Rao (Jana) Doppa, Washington State University, Pullman, WA; Yu Wang, Tsinghua University, Beijing, China
The robotics industry holds enormous promise but development rates are bogged down by increasingly complex software to meet performance and safety requirements in the face of long tail events. Moreover, intelligent robots should adapt in the field to unexpected conditions that may not have ever been observed during design time. Design automation for autonomy has the potential to accelerate the rate at which we overcome these challenges (particularly outside of the autonomous driving sector which throws massive resources at the problem.) In this talk I discuss how the key tools of machine learning, AutoML, simulation, and design optimization have made an impact on systems development for two medical robotics projects - ocular microsurgery and tele-nursing - and will continue to make an impact in other sectors like automated warehouses, service robots, and agriculture.
Presentations include:
;
New Frontiers in Formal and Static Verification
Description:
In this essential session, there will be talks that focus on new explorations of formal techniques and tools by industry giants and research lab. A new approach to use formal analysis to ensure automotive SoC’s adhere to safety standards will be presented. Other presentations focus on architectural analysis, design partitioning and completeness for formal sign-off. Finally, a couple of presentations focus on static verification techniques for reset/power domains and constraint based clock-domain crossing sign-off that circumvent error-prone waiver mechanism.
Presentations:
- Ensuring Completeness of Formal Verification with GapFree: Are we done yet?
- Presenter(s): Ratish Punnoose
- Overlapping Checkers – A Better Substitute of End-to-End Checkers
- Presenter(s): Sumit Kumar Kulshreshtha
- Verifying Reset and Power Domains Together
- Presenter(s): Manjunatha Srinivas, Inayat Ali, Abdul Moyeen, Manish Bhati
- Architectural Formal Sign-Off of Compression System Data Coherency
- Presenter(s): Anuj K. More, Sanjay Bishnoi, David K. Cassetti, Dhruv Gupta, Bhushan G. Parikh, Mark A. Yarch
- LabReplay: Efficient Replay of Post-Silicon Debug for High Performance Microprocessor Designs
- Presenter(s): Arun Joseph, Spandana Rachamalla, Shiladitya Ghosh, Shashidhar Reddy, Pradeep Joy, Sampath Baddam, Samuel Kirchhoff, Joachim Fenkes, Wolfgang Roesner
Chip Design and Cloud: the Good, the Emerging, and the Potential
Description:
In this first-of-its-kind session, you will hear multiple perspectives, from educators to researchers to practitioners on how they have leveraged cloud for their work. Cloud provides a foundational platform to accelerate silicon workflows by providing seemingly limitless capacities. Presentations in this session cover how cloud enabled enhanced delivery of academic courses, technologies to implement hybrid flows, real-world experience from a cloud-based foundry, cloud-native EDA tools or “EDA 3.0”, and finally, workloads that see oversized benefits from cloud.
Presentations:
- Cloud Infrastructure for Remote and Scalable EDA Hardware Training
- Presenter(s)Matthew Morrison, Owain Jones, Kevin Dobie
- The Reality and Opportunities of Semiconductor Design on the Cloud
- Presenter(s)Taeil Kim, Naya Ha, Jongho Kim, Kyungtae Do, Sangyun Kim
- Utilizing the Cloud to Increase Library Characterization Throughput and Reduce Schedule Bottlenecks
- Presenter(s)Kenneth Chang, Dnyanesh Digraskar, Matthieu FillaudWei-Lii Tan
- NEXA: Cloud Native Platform for Collaborative Hardware Logic Design in Step-wise
- Refinement Implementation Flows
- Presenter(s)Arun Joseph, Sampath Baddam, Shashidhar Reddy, Balaji Pulluru, Pradeep Joy, Ajay Gopalakrishnan, Shiladitya Ghosh, Arvind Haran, Anthony Saporito, Matthias Klein, Wolfgang Roesner
- New file system to automatically "spill" workloads across Datacenter and Cloud
Presenter(s)Alok Sinha, Rajeev Prasad, Jasmin Ajanovic
All Routes Lead to Closing Timing
Description:
Timing closure is the final stage of physical implementation, and where some of the most complex challenges can be encountered, creating critical schedule risk. This session covers methods to help ease timing closure, including optimization of routing layer usage, removal of pessimism from margins, quick/accurate parametrized block placement, and accelerated hierarchical ECO generation.
Presentations:
Innovative Solutions for Simulation and Registers
Description:
In this session, you will learn a variety of innovative automation techniques for test/debug, verification and validation of SoC designs.
Presentations:
- Machine Learning Based Efficient Regression Test Framework in SOC Verification
- Presenter(s): Jicheon Kim, Daewoo Kim, Seonil B. Choi
- DIMM Level Verification Methodology for DRAM Custom DFT
- Presenter(s): SeaEun Park, ByeongJun Bae, SeoHa Yang, IJae Kim, YounSik Park, JungYun Choi
- Get more out of your UVM register Layer!
- Presenter(s): Pavan Yeluri, Ranjith Nair
- Unified FW/ASIC Co-Simulation for Earlier and Accelerated Pre-Silicon Testing
- Presenter(s): Elliot Gin, Anthony Cabrera, Joshua Loo, Kiel Boyle, Scott Nelson, Kyle Balston
- Novel end to end Non-coherent access mechanism on X86 SOC
- Presenter(s): Manoj Kumar Munigala, N. Madhusudhan, Surinder Sood, Harshal Mumaikar
Is Your Product Secure? - An IP Driven Approach to Product Security
Presentations:
Embedded Systems! Projects and Solutions
Description:
Embedded systems have become a necessity in every aspect of our daily life. Embedded systems design and deployment pose significant challenges in the areas of compute, power, privacy, security, connectivity, scalability and reliability. DAC Embedded systems track brings together embedded system software developers, IC designers, security experts and product managers to analyze and discuss current and future trends in the embedded systems field. In this Embedded systems session we will be discussing the challenges in security, real-time software design, Machine learning hardware accelerators, and performance modeling software design challenges.
Presentations:
Exploring the New Waves
Description:
Explore topics from multiple domains of system design considerations to debug and integration for bringing your solutions to life. Engage to see if they make a splash with your curiosity.
Presentations:
- Virtual Environment for Developing Reinforcement Learning and Its application for thermal management
- Thermal-aware SOC floorplanning method based on a customized Deep-Q Network algorithm
- Signal Integrity aware HBM3 6.4Gbps interface Channel Optimization
- Presenter(s): Tae Yun Kim, Soo Hyang Jeon, Chan Min JoSung Wook Moon
- Optical and Thermal Simulations for Integrated III-V/Si Heterogeneous Lasers on Silicon Photonics System
- Presenter(s): Stanley Cheung, Antoine Descos, James Pond, Karthik Srinivasan, Stephen Pan, Norman Chang, Di Liang, Raymond Beausoleil
- Systematic Generation and Refresh of Standard Cell Abutment Database
- Presenter(s): Anuradha Ray, Veny Mahajan
- Silicon Debugging Using Function Failure Oriented Path Delay Fault Vectors
- Presenter(s): Keunsoo Lee, Jaehyeon Kang, Sungmin Oh, Yun HeoIlryong Kim, Khader Abdel-Hafez, Girish Patankar, Ruifeng Guo, Tae-Jin JungYong, Joon Kim
Novel Methods for Clocking and Functional Safety
Description:
Clocking continues to be an important aspect of chip development, end to end functional safety is increasingly becoming more important. This session explores novel methods in both of these important aspects of chip design.
Presentations:
- Practical Method for Clock Domain Crossing Using Simulation-Based Path Extraction
- Presenter(s): YoungRok Choi, Hyungjung Seo, So-Jung Park, ByongWook Na, Younsik Park, Jung Yun Choi
- A Novel Clock Gating Design and Verification Methodology to Ensure Safe Power Optimization
- Presenter(s)Hao Chen, Ang Li, Suhas Prahalada, Howard Yang, Miguel Gomez-Garcia, Jonathan E. Schmidt, Leo Sporn
- Automatic Clock Gating and Closed-Loop DVFS for 4nm Exynos Mobile SoC Processor
- Presenter(s): Jae-Gon Lee, Wookyeong Jeong, Jaeyoung Lee, Byung Su Kim, Se Hun Kim, Young Duk Kim, Youngsan Kim, Yonghwan Kim, Sung Hoon (Ryan) Shim, Byeongho Lee, Jong-Jin Lee, Hoyeon Jeon, Younsik Choi, Joonseok Kim
- Accelerating mutation coverage measurement by using concurrent fault simulator
- Presenter(s): Kota Sakai, Kenichi Otsuka, Fumitaka Fukuzawa, Shintaro Imamura
- Analog Fault Simulation for Automotive Sensor Designs
- Presenter(s): Soohyun Kim, Yun Heo, Seokyong Park, Seongyeop ParkIlryong Kim, Sungjin Park, Kwangsoo Seo
- Efficient Data Exchange Towards Faster Functional Safety Development
- Presenter(s)Shivakumar Chonnad, Vladimir Litovtchenko
Performance, Aging, Reliability - Key Analyses
Description:
Learn and engage on managing performance - aging, power delivery and squeezing the last bit of performance while saving on area
Presentations:
- Efficient System PDN Analysis Methodat Pre-Layout Stage
- Presenter(s): Seungki Nam, Jungil Son, Jeewon Kwon, Sumant Srikant, Haemin Lee, Sungwook Moon
- Aging aware Static Timing Analysis
- Presenter(s): Sangwoo Han, Chirayu Amin
- Machine Learning based IR Drop Prediction on ECO Revised Designs for Faster Convergence
- Presenter(s): Sashank Nishad Santanu Kundu, Manoranjan Prasad
- Simultaneous Design Methodology of High Speed & High Density Cell Libraries Using Two Different Rows in Single Design
- Presenter(s): Dayeon Cho, SungOk Lee, Sangdo Park, Hyung-Ock Kim, Sungyoul SeoIngeol Lee, Sangyun Kim
Solidifying your SOC beyond Design
Description:
Does a solid design in itself guarantee solid end product? With SOC Design methodologies well understood, often times teams face challenges in terms of having robust Silicon due to power/performance, test coverage and reliability related issues. In this session, experts will talk about some of the key factors that can be considered during design phase of the Chip to solidify the Silicon. We will look at techniques used by some of the experienced engineers to improve effects of aging and reduce variability challenges, ways to improve power and performance of your designs and key considerations while integrating Mixed-Signal IPs.
Presentations:
Solving Power Challenges at the Front End
Description:
This session covers various low power design techniques and power reduction methodologies to achieve most optimal power budgets. In this session, audience will learn about recent innovations in low power domain related to coverage mechanism, workload management and advancement in the field of power and performance.
Presentations:
Tune in to the Clocks!
Description:
Clock design continues to be an important aspect of the overall chip design. In this session, you would come across some novel techniques used to resolve challenges in clock design during chip development.
Presentations:
;
Autonomous Robot Design: How can EDA help?
Organizer:
- Iris Bahar (Brown University)
Moderator:
- Iris Bahar (Brown University)
Panelists:
- Hadas Kress-Gazit Cornell University
- Sonia Chernova, Georgia Tech
- Sabrina Neuman, Harvard University
- Shaoshan Liu, Perceptin
Quantum Computing: An Industrial Perpective
Organizer:
- Michael Niemier (Notre Dame)
Moderator:
- Robert Wille (Johannes Kepler University Linz)
Panelists:
- Leon Stok, IBM
- Krysta Svore, Microsoft
- Austin Fowler, Google
Homomorphic Computing as a foundational technology: Theory, Practice, and Future Business
Organizer:
- Yiorgos Makris, UT Dallas
Moderator:
- Mihalis Maniatakos, New York University
Panelists:
- Kurt Rohloff, Duality
- Kim Laine, Microsoft Research
- Ingrid Verbauwhede, KU Leuven
- Shafi Goldwasser, MIT
Environmentally-Sustainable Computing
Organizer:
- Carole-Jean Wu, Facebook & Arizona State U.
Moderator:
Panelists:
- Srilatha (Bobbie) Manne, Facebook
- Karen Strauss, Microsoft
- Fahmida Bangert, ITRenew
- David Brooks, Harvard
- Andrew Byrnes, Micron
*If you've previously registered for 58th DAC, please use the guide below for information of what's included in your registration.
.png?ver=Epx1mVy_emQz2bFl4yQ7mg%3d%3d)